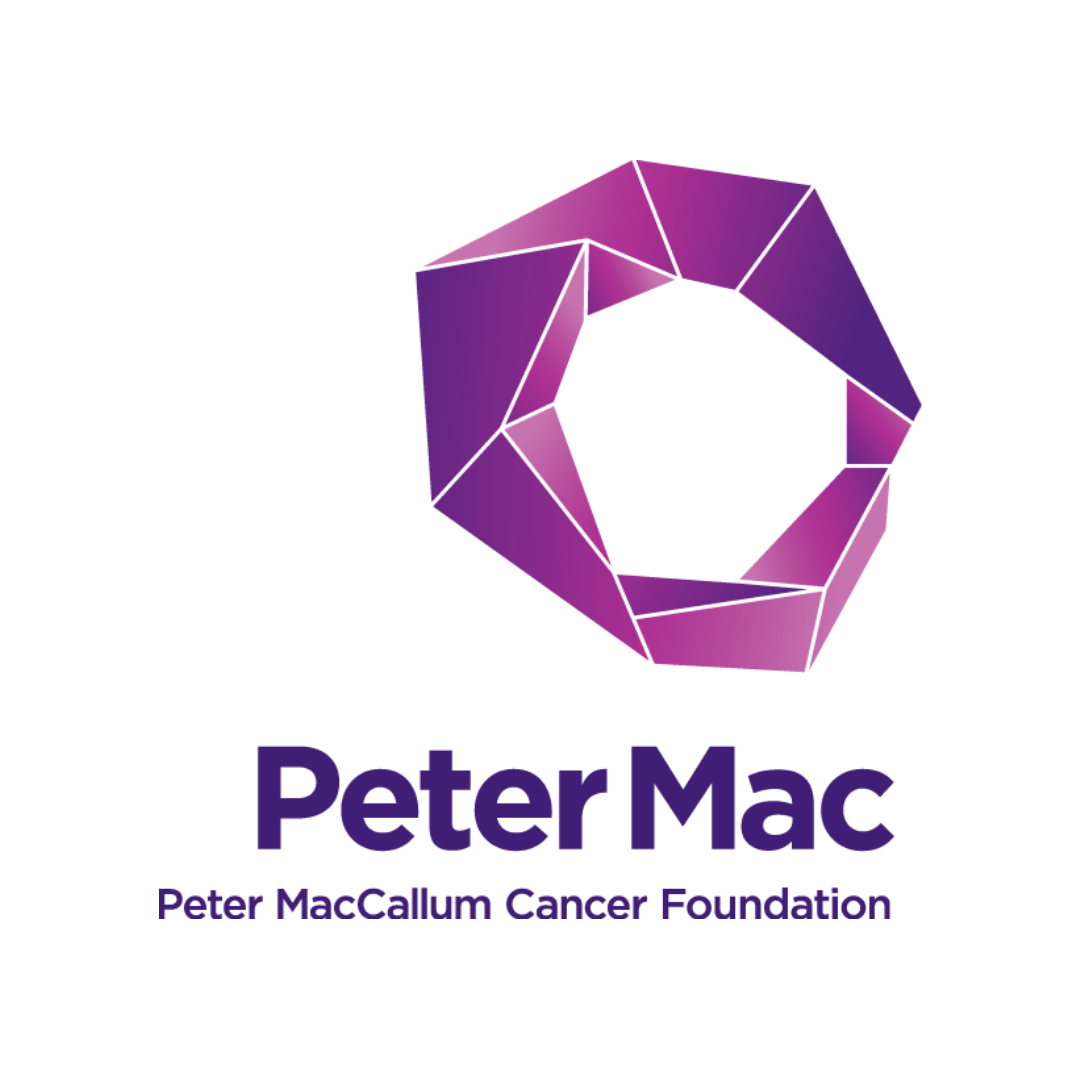
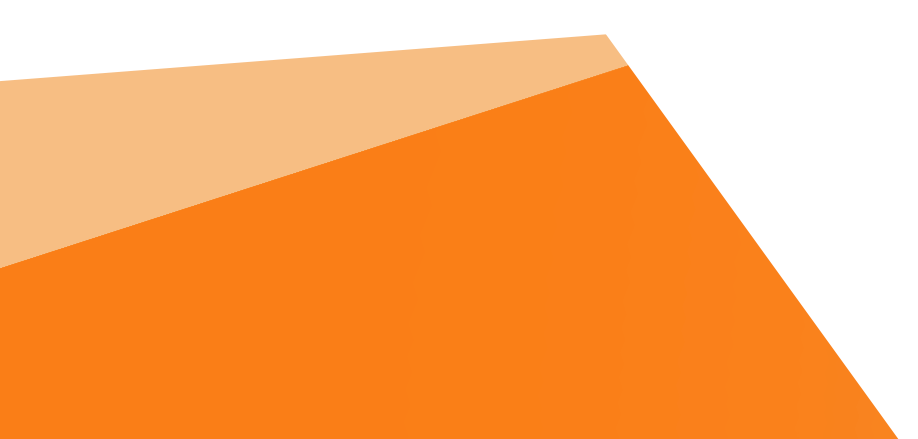
Why Targeted Drugs and Therapies Demand Novel Biomarkers for Assessing Tumour Burden
Cancer treatment is determined by the type of cancer and its stage. If caught at an early stage, treatment options include surgery or radiotherapy. Chemotherapy, drugs that potentially kill cancer cells, is necessary at a later stage when the cancer is more advanced or has spread. Chemotherapy is delivered systemically and acts with limited specificity in the body: cancer cells and healthy cells are affected and therefore, chemotherapy can result in severe side effects.
Today we see the rise of targeted cancer therapies and high-precision imaging
Over the last few decades several novel treatment modalities have become an important part in the fight against cancer.
Targeted cancer drugs work by ‘targeting’ differences of a cancer cell on a molecular level. Popular targets include those important for cancer growth and progression. Targeted drugs for example stop cancer cells from dividing, encourage the immune system to find and kill cancer cells or stop cancers from growing blood vessels. They are delivered systemically and can still have side effects, but, are generally less severe than chemotherapy.
Stereotactic ablative body radiotherapy (SABR) is a novel high-precision treatment modality. Radiotherapy beams originate from different positions around the body and are calibrated by medical imaging. As a result, the tumour receives a high dose and the surrounding tissues a lower dose. Careful treatment planning and the inherent high-precision of the technology allows that the radiotherapy field covers the tumour while avoiding healthy tissue as far as possible. This reduces the risk of side effects.
Effects to the Tumour Morphology
While novel cancer treatments are different in their delivery and mode of action, they share a common motivation and morphological tumour response.
Innovative therapies are specific by focusing on local control of the tumour and thus, lower the risk of side effects. Besides being effective in cancer cell death, they do not necessarily result in tumour morphological change: a residual non-viable tumour architecture can remain for a sustained period post-treatment.
Broadly speaking, novel therapies result in a complex tumour remnant that complicates the assessment of changes in tumour burden.
Cancer Therapy Validation
New drugs and therapies require a thorough validation showing treatment success. The Response Evaluation Criteria in Solid Tumours (RECIST) provides a validated and consistent methodology to evaluate the activity and efficacy of new cancer therapies in solid tumours: RECIST determines the tumour diameter on cross-sectional computed tomography (CT) or magnetic resonance imaging (MRI) scans to assess changes in tumour burden.
In January 2017, the RECIST working group has published on the continued relevance of RECIST guidelines and on the challenge to maintain RECIST as a standard for the assessment of tumour burden in clinical trials. The authors have acknowledged that “…maintaining the validity and relevance of RECIST as a standard evaluation approach is challenging, in particular to maintain a balance between its specificity and generalizability…” Furthermore, the authors noted that the RECIST linked data collection in clinical trials limits the utility of existing databases for the validation of new evaluation approaches to tumour response.
Modifications to Adjust to Novel Therapies
It is critical to note that RECIST version 1.1 remains the only general tool that provides harmonization of tumour response assessment.
However, the working group and a large body of research brings to light the acute need for new imaging modalities, assessment methodologies and updated criteria to support the development of the new classes of treatments. As a response to this need, the immune Response Evaluation Criteria in Solid Tumours for cancer immunotherapy trials (iRECIST) has been developed and validated.
Advanced Imaging and Analysis Methodologies
Recent research has shown that advanced imaging and Artificial Intelligence (AI)-powered analysis provide detailed insight into therapy related tumour microenvironmental changes and are able to predict RECIST outcomes.
MRI poses a promising alternative to evaluate tumour response and is currently rated as equivalent to CT by the American College of Radiology for solid tumours. Multi-parametric MRI (mpMRI) is a sequence of anatomical and functional MRI scans. Several solid tumour trials have successfully implemented mpMRI as an effective explorative endpoint; mpMRI’s ability to predict pathological response is considered as an important advantage over CT.
IAG, Image Analysis Group collaborates with Peter McCallum Cancer Center
IAG’s recent collaborative clinical trial in stereotactic ablative body radiotherapy (SABR) in renal cell cancer (RCC) has implemented a mpMRI protocol.
IAG’s expert team designed and deployed a dynamic contrast enhanced (DCE) MRI and diffusion weighted MRI (DWI) as part of a mpMRI imaging protocol to provide earlier evidence of response (Reynolds et al., 2018).
Resulting quantitative imaging biomarkers have shown to be predictive to RECIST-based assessments.
Together, with our scientific collaborators, IAG’s team brought forward novel imaging biomarkers that have shown significant correlations between the tumour volume change and changes in advanced perfusion imaging biomarkers.
The figure to this article shows colour coded parametric maps before and after therapy. More in the full article.
Full Article: Diffusion weighted and dynamic contrast enhanced MRI as an imaging biomarker for stereotactic ablative body radiotherapy (SABR) of primary renal cell carcinoma
About IAG, Image Analysis Group
IAG, Image Analysis Group is a unique partner to life sciences companies, leading AI-powered drug development and precision medicine. IAG leverages expertise in medical imaging and the power of Dynamika™ – our proprietary cloud-based platform, to de-risk clinical development and deliver lifesaving therapies into the hands of patients much sooner. IAG provides early drug efficacy assessments, smart patient recruitment and predictive analysis of advanced treatment manifestations, thus lowering investment risk and accelerating study outcomes. IAG bio-partnering takes a broader view on asset development bringing R&D solutions, operational breadth, radiological expertise via risk-sharing financing and partnering models.