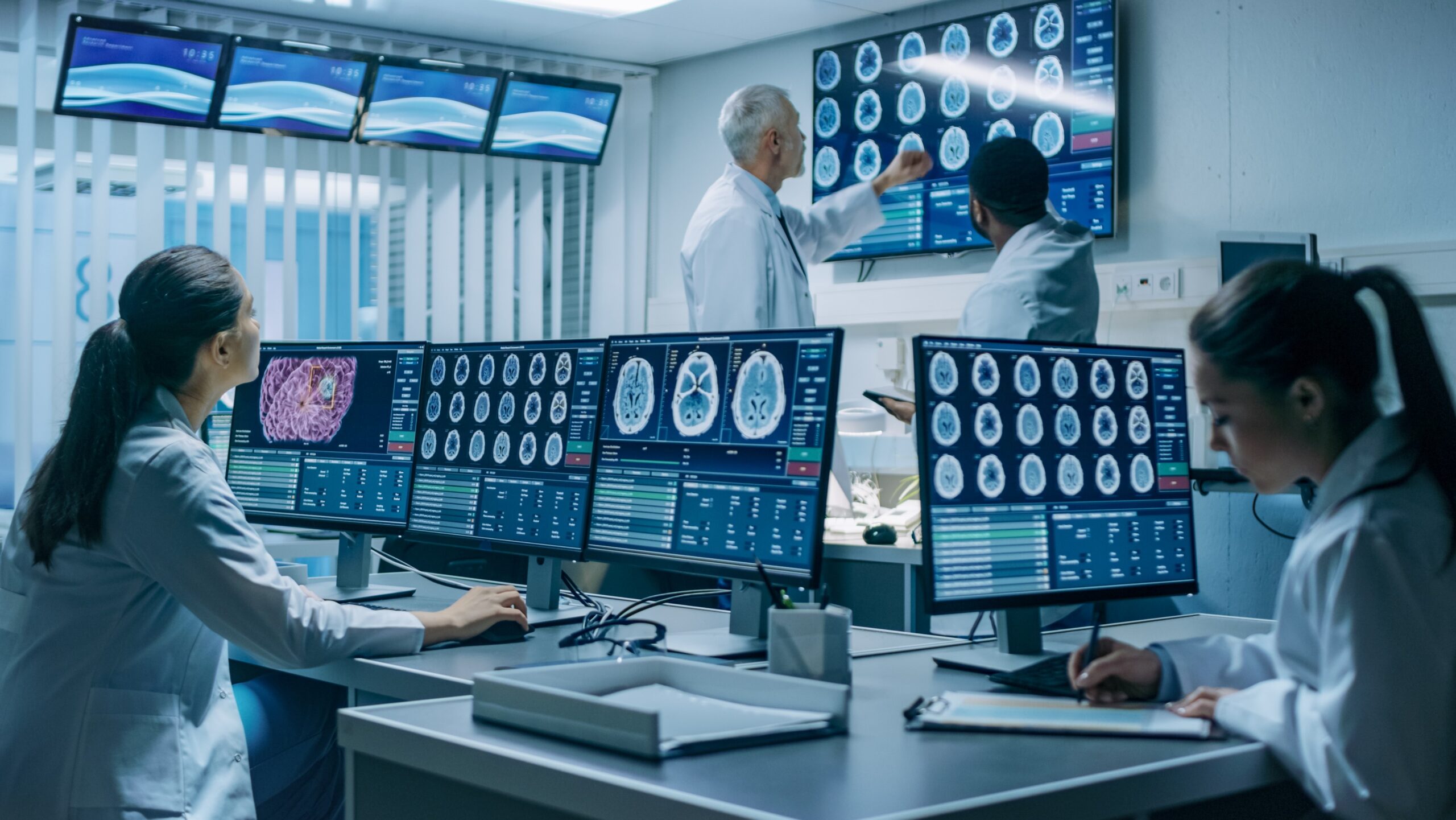
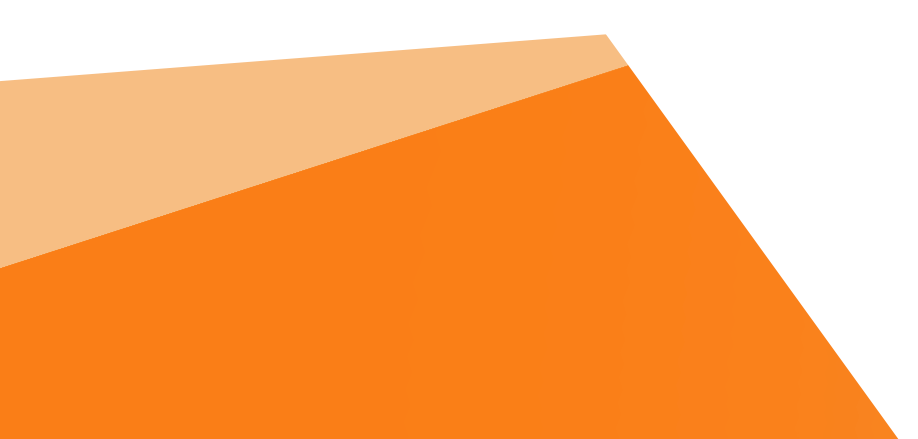
Machine Learning & Radiomics for Oncology Drug Development
Success of oncology drug development lays in early insight into tumor signature or the microstructural phenotype that sheds light on the key functional processes that determine tumor behavior, such as vasculogenesis, hypoxia, fibrosis, necrosis, immune cell recruitment and tissue infiltration.
Linking tumor phenotype and mechanism of action of a novel drug helps answering key clinical questions, such as primary tumor aggressiveness, metastatic potential and tumor response to various therapies.
These questions cannot be fully answered through the conventional imaging modalities and established approaches in drug development.
However, a new way of thinking comes through the process of transferring the radiology interpretation, knowledge and skill set from humans to machines in a way that they can see more, process more, and have deeper insights into what the disease is, how it behaves and how it might respond to therapeutic intervention [1].
This process is called radiomics.
One of the earliest applications of radiomics were in the field of oncology, where radiomics driven biomarkers help our understanding of the molecular and microstructural processes, brining critical supportive information as exploratory endpoints for clinical trials.
There is good reason to be excited about radiomics and how it can enhance our understanding and management of cancer. It leverages the power of machine learning to classify the several hundreds of extracted features clustered to quantify biomarkers.
However, to ensure reliability of the answers, especially in early drug development, there is a pressing need to adopt structured and data driven approach to using radiomics.
The first wave of literature in this field heavily focused on the plethora of features that could be extracted and then classified to indicate key pathophysiologic milestones in disease progression [2-5]. This wealth of information was poised to improve the sensitivity of cancer diagnostic and prognostic approaches; however, the trade-off is the decline in specificity. The next wave of radiomics literature attempted to validate the accuracy, repeatability and reproducibility of this methodology, and the results were not as convincing [6,7].
This pattern of hype over a new modality or methodology that seems to significantly improve sensitivity but then fails to meet the stringent criteria of accuracy and inter-reader and inter-center reproducibility is not new. Genomics has gone through a similar cycle!
In order to make the most from the radiomics, we need to improve the specificity of radiomics as a methodology.
Our current research with support with many academic and clinical collaborators is focused on
- defining the clinical question at hand,
- understanding the intricate pathophysiologic processes, and
- carefully selecting which radiomics features are best able to answer that question.
This calls for a highly nuanced approach to radiomics based on a clear understanding of the clinical manifestations of disease and the cellular/subcellular processes that drive them.
A number of studies have already shown radiomics to be repeatable – van Velden, et al. showed that there is high repeatability of using radiomics assessment for FDG PET/CT in patients with Non-small cell Lung cancer, comparable or even better than the standard-of-care standardized uptake value (SUV) measurements [8], and Schwartz, et al. showed that radiomics approaches are reproducible [9]. Some of the latest studies have shown promising results taking radiomics as the ultimate non-invasive microstructural and functional decoder of cancer with very specificity levels [10-12].
Radiomics biomarkers are indeed powerful but using this approach like the Computer-aided diagnosis black box, will be fruitless or at least redundant.
At IAG, we are solving many technical and statistical challenges using standardized workflow and multi–expert input into study designs and data processing. Success of the treatment specific and the bespoke radiomic approaches is in targeting the exact modalities, post-acquisition reconstructions, and objectively selecting radiomics features for s specific disease to answer the precise clinical question at hand. Rigorous statistical validation and plentiful real world experience of highly targeted applications thereof is needed in order to usher it into the clinical realm where it can be used with a high level of confidence.
As the next step through our DYNAMIKA platform, we are bringing this exactment into multicenter, randomized clinical trials, where the ground reality dictates significant variations in patient preparation, image acquisition, post-acquisition reconstruction and reader biases.
We believe that widespread acceptance of radiomics as a noninvasive and reliable method can ultimately meet or ideally surpass the validity of biopsy-based tissue analysis approaches, especially as more and more studies show that radiomics is indeed more or as accurate.
References:
- Radiomics: Images Are More than Pictures, They Are Data. Gillies RJ, Kinahan PE, Hricak H. Radiology. 2016 Feb;278(2):563-77. doi: 10.1148/radiol.2015151169. Epub 2015 Nov 18.
- Beyond imaging: The promise of radiomics. Avanzo, Michele et al. Physica Medica: European Journal of Medical Physics, Volume 38, 122 – 139
- Decoding tumour phenotype by noninvasive imaging using a quantitative radiomics approach. Aerts HJ, Gillies RJ11, Lambin P, et al. Nat Commun. 2014 Jun 3;5:4006. doi: 10.1038/ncomms5006.
- Chicklore, S., Goh, V., Siddique, M. et al. Eur J Nucl Med Mol Imaging (2013) 40: 133. https://doi.org/10.1007/s00259-012-2247-0
- Radiomics: Extracting more information from medical images using advanced feature analysis. Lambin, Philippe et al. European Journal of Cancer, Volume 48, Issue 4, 441 – 446
- Applications and limitations of radiomics. Yip S, Aerts H. Physics in Medicine & Biology, Volume 61, Number 13
- Technical Challenges in the Clinical Application of Radiomics. Shaikh F, Aerts H, et al. JCO Clinical Cancer Informatics 2017 :1, 1-8.
- Radiomics analysis of pre-treatment [18F]FDG PET/CT for patients with metastatic colorectal cancer undergoing palliative systemic treatment. van Helden EJ, Vacher YJL, van Wieringen WN, van Velden FHP, Verheul HMW, Hoekstra OS, Boellaard R, Menke-van der Houven van Oordt CW. Eur J Nucl Med Mol Imaging. 2018 Dec;45(13):2307-2317. doi: 10.1007/s00259-018-4100-6. Epub 2018 Aug 9.
- Reproducibility of radiomics for deciphering tumor phenotype with imaging. Zhao B, Tan Y, Tsai WY, Qi J, Xie C, Lu L, Schwartz LH. Sci Rep. 2016 Mar 24;6:23428. doi: 10.1038/srep23428.
- Can radiomics personalise immunotherapy? El Naqa I, Ten Haken RK. Lancet Oncol. 2018 Sep;19(9):1138-1139. doi: 10.1016/S1470-2045(18)30429-7. Epub 2018 Aug 14. Lancet Oncol. 2017 May;18(5):e266-e273. doi: 10.1016/S1470-2045(17)30252-8. Epub 2017 Apr 26.
- The future of personalised radiotherapy for head and neck cancer. Caudell JJ1, Torres-Roca JF1, Gillies RJ2,et al.
- E Deutsch, N Paragios, Radiomics to predict response to immunotherapy, bridging the gap from proof of concept to clinical applicability?, Annals of Oncology, , mdz150, https://doi.org/10.1093/annonc/mdz150