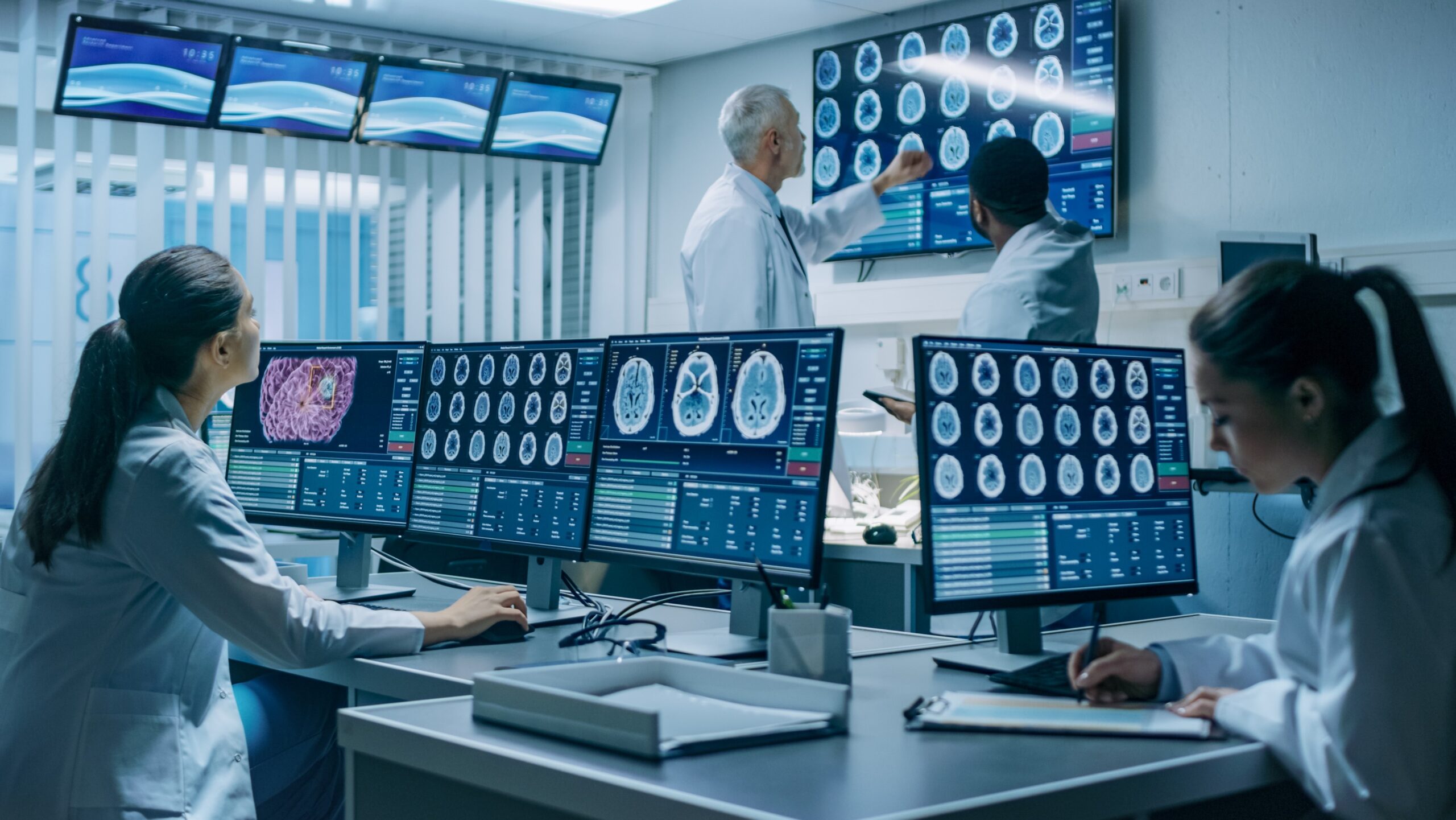
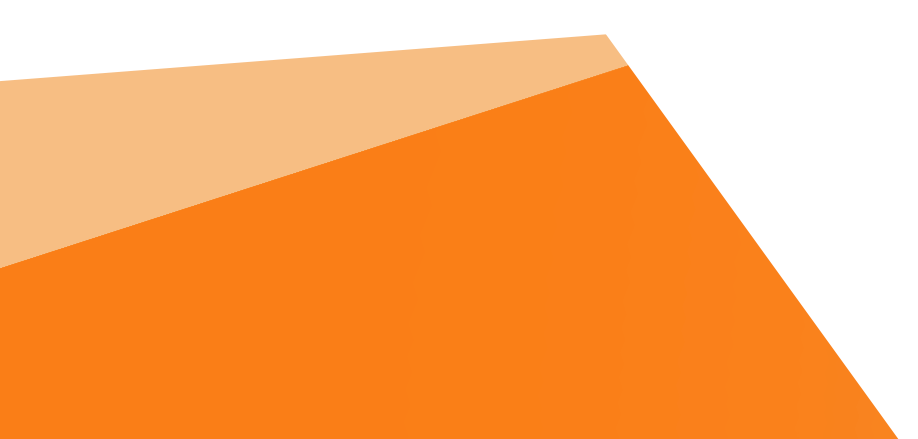
3 Types of Artificial Intelligence (AI) to Impact Clinical Research in the Next 3 Years
Generally, research studies recommend looking at AI through the lens of business capabilities rather than technologies. There are three types of AI being deployed by life science businesses that can provide a framework for improving efficiencies. Very broadly, AI can support 1) automation of business processes, 2) gaining insight into data through data analysis, and 3) making critical decisions based on the large volumes of data and thus impacting overall business through the big data analysis and engagement with the customers, patients, suppliers and employees.
-
Process Automation / Focus on Efficiencies
This type of AI relates to automation of what we can broadly call ‘back-office’ function and it is deployed through more efficient handling of digital and physical tasks using technology.
Such tasks may include
- Transferring the data from a site to a storage or transferring the data from a call or email into a record storing system.
- Reconciling failures in systems or data by combining and automatically checking information in multiple systems and multiple document types.
- Reading radiology or clinical reports through Natural Language Processing techniques to extract provisions, data points, and ultimately making conclusions.
This type of AI is the simplest and least expensive to implement. In the clinical research industry, data transfer, data quality control, and data management processes feel somewhat archaic. It inherits a lot of inefficiencies founded in utilization of manual labour, outdated technologies and hardware. The efficiencies and quality of the outcomes can easily be improved through the use of the Cloud Enterprise solutions.
Cloud solutions, such as IAG’s DYNAMIKA , allow companies to create an efficient infrastructure between the suppliers of data (sites or hospitals), customers (biotech or pharma companies) and data analysis function of the business.
The infrastructure allows oversight of the performance of each supplier, ensures full transparency of the overall delivery, assists each stakeholder in communicating their needs, and requests but most importantly allows the customer to make fast decisions based on real data.
This type of AI is what we would call a ‘’low hanging fruit’’ that can bring immediate returns.
It is also the least ‘’smart’’ in a sense that these applications are not programmed to learn and improve, though our developers at IAG are thinking through how to add more intelligence and learning capabilities into DYNAMIKA.
Examples of such intelligence would include automating a link between a radiology reader looking at CT or MRI and measuring a tumors size and the scoring system called RECIST or a radiologist looking at MRI of a rheumatoid arthritis patient hand and RAMRIS form. Other companies are coming up with a way to extract clinical information from the radiology or pathology report and input it directly into the trial database.
GE for instance used AI to integrate supplier data and saved $80m in its first year by eliminating redundancies in negotiating contracts, which were previously managed at the business unit level. Similarly, one of the largest banks used AI to match the contracts from different suppliers, identifying tens of thousands of products and services which were not supplied.
-
AI Driven Data Analysis / Focus on Precision of Go / No Go Decision
This type of AI is often feared by specialists and referred to as better decision making or replacing a human in the making a decision process. Strictly speaking, it is not true. The AI driven methodologies in clinical research are generally designed to aid the human to make a decision. We can broadly separate AI methods into semi or fully automated.
An example of fully automated AI driven method would be if someone takes an MRI scan and when the scan gets processes by the software we know if the patient has a particular disease or not.
A majority of the methods are semi-automated and will require either the initial input or the final decision made by an expert, however the AI algorithm will handle everything in between in a fully automated manner.
We use semi and fully automated methodologies for the assessment of medical images such as MRI, CT, Xray, US. We use these alongside with the human assessor who would assess pre-processed imaging data instead of the raw data. The decision made with the use of AI will be made faster and be potentially more accurate. We will also be able to look deeper into the data, extracting valuable information which might not be noticed by a human eye from the raw images.
At the very beginning of clinical research, all data was analysed by experts and such ways are currently referred to as the ‘state of the art’. The industry has moved to bring automation in pathology, assay analysis and of course image analysis, which perhaps is the most visual of them all.
It is important to note that an expert and the machine will most likely make the same decision. This implies that we are speaking about the actual expert and a well-designed robust algorithm.
The advantage in using AI driven techniques is that it would allow to recognize much finer details or pick up more subtle differences, which in turn will allow achieving statistical significance on smaller patient populations or making more robust decision (statistically speaking), which often makes all the difference.
We wrote several case studies of our companies using the advanced imaging and machine learning in several therapeutic areas. These are being constantly updated and worked on, but some examples can be found here
This type of AI is easy to implement in a fragmented manner or alongside of something which might look more familiar, such as a state-of-the-art method. The use of such AI in decision making in phase III studies as a methodology for extraction of biomarkers will require consensus of regulatory bodies and several groups are working towards making this possible. However, in early phase studies where it is the company’s decision and such decision needs to be based on the comprehensive and robust data, this type of AI will bring high ROI by ensuring less failures in the later phase trials.
-
AI and Insight into the Data /Focus on Business Decisions
This type of AI is used to detect patterns in vast volumes of data and interpret their meaning.
Here you will find a blend of machine learning techniques and true artificial intelligence. Examples of AI would be the prediction of a particular cancer type based on a combination of genes, automation of personalized targeting for success of a particular therapy, choosing the right dosing based on the treated patient statistics and much broader.
In related markets, this type of AI will inform the insurer with more accurate and detailed actuarial modelling, inform drug discoverer of most likely combinations to succeed and fail, intelligently influence the design of your next trial based on the competitive intelligence, and utilize endpoints and patient populations.
An example of big data analytics would be a project where a www.clinicaltrials.gov and all relevant publications got assessed by a machine to collect information on a particular treatment to inform which sub-populations would most likely benefit from it.
AI driven insights are very different from the traditional analytics as they are much more data intensive and detailed and they utilized a particular model which gets better with more data and time. With the data obtained in any single interaction, the model can improve on its performance generating more reliable results.
Such AI is typically used to improve performance or decisions only a machine can do.
Other examples of such AI type would include
- Intelligent agents that offer 24/7 customer service addressing issues like passport requests. Technical support to refereeing a customer to the right specialist
- Health treatment recommendations systems that help providers create customized care plans that take into account individual patient history, previous treatments and patient analytics.
- A biotechnology or pharma company combining all their early stage data to determine the next indication where a drug is more likely to succeed.
This type of AI is the most expensive to implement as it would require the first 2 types to be in place for added efficiencies. This definitely is not a low hanging fruit and will require consensus and readiness to change from all levels of the organization.
It is the author’s personal view that it is very actual and critical for smaller biotechnology companies with platform technologies who are at the early stages of the development or just got their first success. Capitalizing on this success with the use of the best technologies will yield long term benefits and sustainable growth.
To conclude, AI, in either form provides a powerful mechanism of improving the business performance and decision power. However, before embarking on any AI initiative, the companies must understand which technologies perform what type of tasks and the strength and limitations of each. In order not to waste valuable time and resources, it is important to set the right expectations from the very start. For instance, rule based systems can make decisions but are not going to learn; machine learning driven analysis can outperform a human when given the right data but might fail if the variation is too significant. The black box technology company should present sound validation and certification of quality of the outcomes and so on. If you don’t have data science capabilities in house, you need to build an ecosystem to enable seamless data flow to ensure success of the entire operation.
If you read till the end and would like to join likeminded individuals, please join our Linkedin group ‘AI and Machine Learning in Clinical Research’ or contact me for more information (olga.kubassova@localhost).
References
BOOKBINDER, M. (2017, September 29). The Intelligent Trial: AI Comes To Clinical Trials. Retrieved April 11, 2018, from http://www.clinicalinformaticsnews.com/2017/09/29/the-intelligent-trial-ai-comes-to-clinical-trials.aspx
BRYNJOLFSSON, E., & MCAFEE, A. (2018, February 01). The Business of Artificial Intelligence. Retrieved April 12, 2018, from https://hbr.org/cover-story/2017/07/the-business-of-artificial-intelligence
RONANKI, R., & DAVENPORT, T. H. (2018). Artificial Intelligence for the Real World. Harvard Business Review, 108-116. Retrieved from https://hbr.org/2018/01/artificial-intelligence-for-the-real-world.
Author:
Olga Kubassova, PhD, CEO and Founder of IAG
Biography: Dr. Olga Kubassova is a CEO of IAG, mathematician by background with many years of effective asset positioning and implementing strategies for clinical development, growth, partnering and exit. Her expertise is in identifying and integrating novel approaches to support fast decision making on the efficacy of new therapeutic assets. She broadly leverages her background in computer science, mathematics and imaging to support companies, developing novel technology platforms and decision- making systems. Olga is a renowned healthcare innovator, author of over 40 papers, book chapters and books, speaker and biotech investor, with passion for improving people’s health.