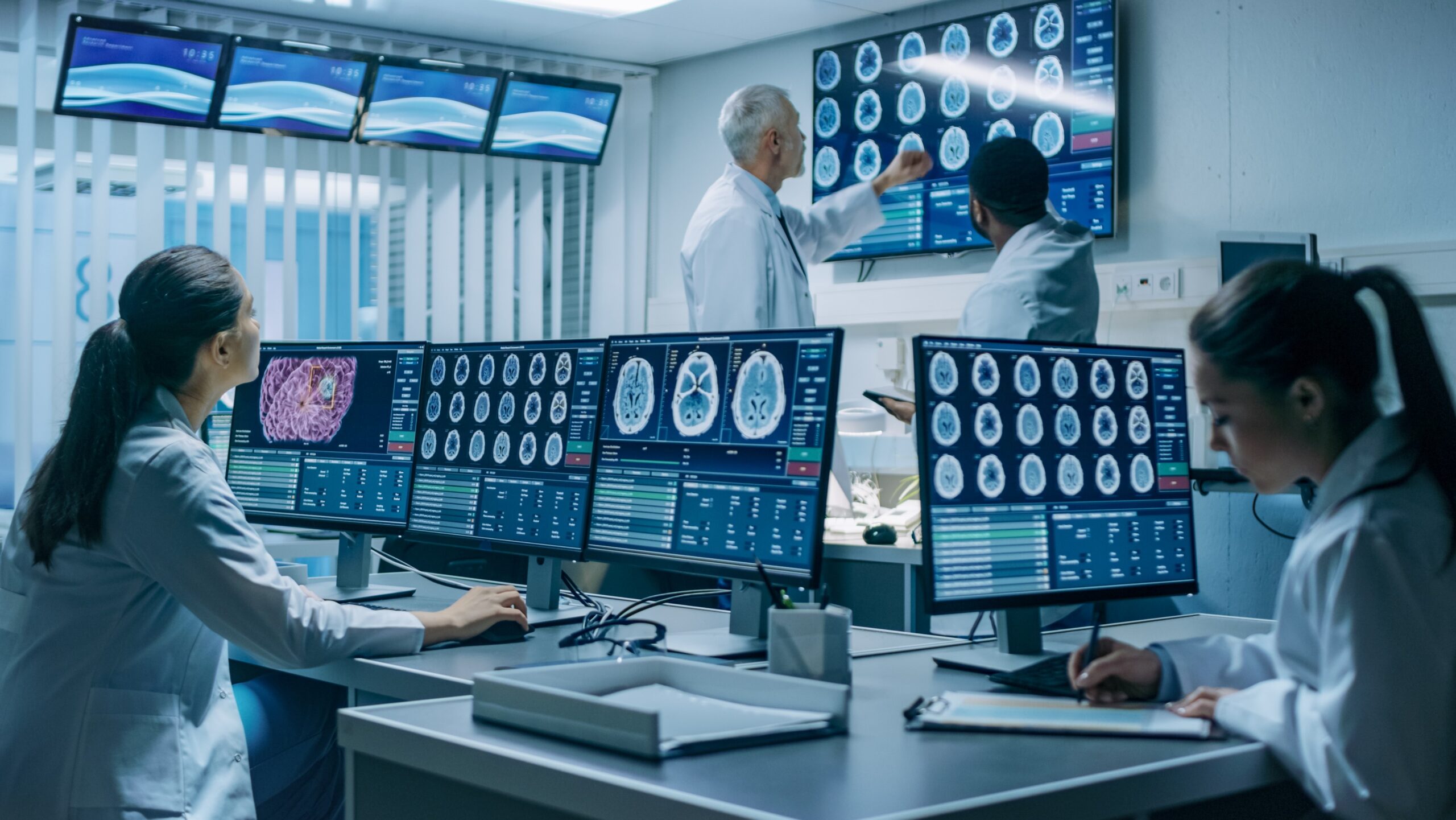
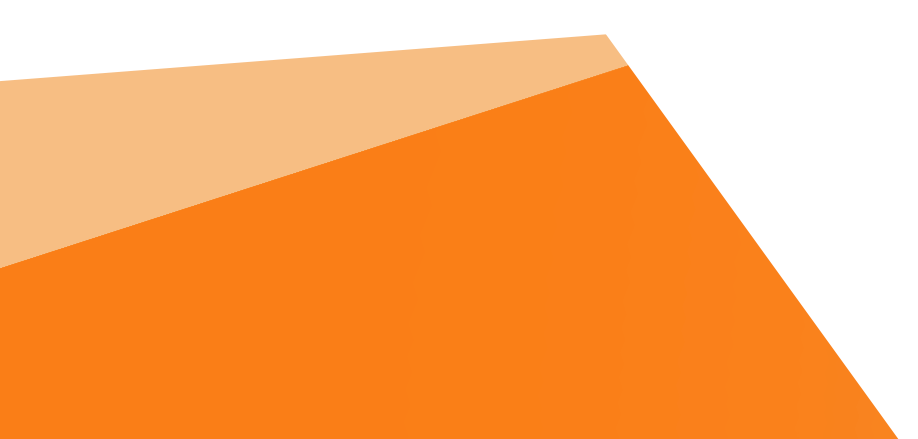
AI & I: Reflection on AI in Drug Development
Artificial Intelligence (#AI) refers to the ability of a computer to perform tasks commonly associated with intelligent beings, such as to correctly interpret data, to learn from it and to use these learnings to achieve specific goals through intelligent adaptation.
The definition of what constitutes AI depends on the goal that is trying to be achieved within an AI system. The most AI systems fit into: Strong – system that thinks exactly like humans do; Weak – system that do not figure out how human reasoning works (IBM’s Deep Blue); In-between – system that uses human reasoning as model, but not necessarily the end goal.
A system that uses human reasoning as model, but not necessarily the end goal is the most exciting AI system, as here the System produces novel insights to provide better services or products as oppose to a replica of the human mind.
Machine Learning (#ML) is an application of AI that provides systems the ability to learn and improve from experience without being explicitly programmed. These look for patterns in data, and through feedback loops, learn and make better decisions in the future based on the examples that we provide.
ML can be categorized broadly as supervised or unsupervised and generally fall into the following categories:
Supervised Machine Learning Algorithms – We have input variables and an output variable, and you use an algorithm to learn the mapping function from the input to the output with training data. The aim is to approximate the mapping function so that when we have new input data, we can predict the output. variables for that data.
Unsupervised Machine Learning Algorithms – information used in training data is neither classified nor labelled. Unsupervised learning studies how systems can infer a function to describe a hidden structure from unlabelled data. The system doesn’t figure out the right output, but it explores the data and can draw inferences from datasets to describe hidden structures from unlabelled data.
Semi-Supervised Machine Learning Algorithms- fall somewhere in between supervised and unsupervised learning, since they use both labelled and unlabelled data for training – typically a small amount of labelled data and a large amount of unlabelled data. The systems that use this method are able to considerably improve learning accuracy.
How AI Can Optimize Drug Development?
The cost of discovering and commercialising a new drug did not go down in decades, taking an average of 14 years and costing up to $2,5bn. Acting across multiple sectors, AI has the ability to not only power research and development, but also provide efficiencies across manufacturing, sales, marketing and procurement.
Looking at the 5-year agreement between Novartis and Microsoft, Novartis CEO Vas Narasimhan notes the potential of “[scaling] the technology across the value chain of the company, that will, I hope, lead to a significant differentiation over time”.
Within the Life Sciences industry, there are three areas in which AI is currently providing a framework for improving efficiencies these stand to support;
1) Automation of businesses processes;
2) Insight into data through data analysis;
3) Decisions based on analysis of large data sets and engagement across multiple stakeholders.
The 1956 Dartmouth Summer Research Project highlighted complex information processing as one of key features of AI in the coming years.
In 2019, this type relates to automation of what we broadly call ‘back-office’ function and allows for automation of business processes. Providing efficient handling of digital and physical tasks using technology.
Whilst this type of AI is the simplest and least expensive to implement, it can offer huge improvements in efficiency in data management and storage from day 1 of implementation.
In the Clinical Research Industry, specifically in what IAG, Image Analysis Group are very good at – handling large volumes of images within a complex environment of a clinical trial, this type of AI allows for efficient data transfer, automated data quality control and optimization of the data management processes.
It is critical to have the right system to make the most out of AI, such as our DYNAMIKA the Enterprise Cloud Platform. Only a few years back it was perfectly acceptable to see a lot of inefficiencies founded in utilization of manual labour, outdated technologies and hardware. However, the cloud based solution, like
DYNAMIKA now allows bio-pharma companies to operate within an efficient infrastructure in the supply chain, with data controls allowing for full transparency of required data available to each stakeholder in real-time 24/7.
The integrated automation of the data management and centralized processes allow each stakeholder to make fast, well-informed decisions driving up efficiencies and keeping costs low.
In clinical research studies, examples of AI intelligence include automating a link between a radiology reader measuring tumour burden on a CT image and the scoring system RECIST, or in the case of rheumatoid arthritis an MRI of a patient’s hand and the RAMRIS form. Other ways of automation include directly extracting clinical information from a pathology/radiology report and uploading to a trial database on a cloud platform in real time, some improvement from the current practices, which can include sending data across continents by CDs and floppy disks!
Where AI can differentiate from conventional human thinking is through novel, non-human insights, derived from otherwise robust data.
This type of AI is often feared by specialists as replacing a human with a machine, which would make a better decision. The AI driven methodologies in clinical research are generally designed to aid the expert when making a decision.
Whilst it is possible to envisage a situation where decisions are fully automated in the case of Yes/No decisions i.e An AI driven method of assessing whether someone who takes an MRI scan has a particular disease. The future of these technologies lies in semi-automated methods with input, oversight and decision making by an expert.
This is of particular importance in the field of oncology and immunotherapy treatment.
Conventional approaches in cancer drug development aim to show drug efficacy based on the assumption that morphological changes are significant. Imaging modalities such as CT in combination with RECIST-based scoring methods are commonly applied. Whilst these can demonstrate drug efficacy in late clinical trials, they fail to take into account changes in the tumour microenvironment (critical in evaluating immunotherapies).
This can result in patients on ineffective treatments for too long, or worse, patients removed from a trial following pseudo-progression, an increase in the size of tumour burden, as a result of immune cell infiltration, in a response to treatment.
Using manual scoring methods such RECIST, this can be taken to mean progressive disease, resulting in patients being taken out of the trial.
Pseudo-progression does not represent disease progression, it often is a marker of longer survival, presumably because it represents a robust response to treatment. Advanced Imaging & AI are at the forefront of supporting the development of advanced therapies.
The advantage in using AI driven techniques is that it would allow to recognize much finer details or pick up more subtle differences, such as infiltration of immune cells.
AI combined with advanced imaging can generate novel imaging biomarkers, carefully designed for a specific drug’s mode of action, resulting in surrogate endpoints that have the potential to predict treatment response early.
Across Drug Development, the use of novel imaging biomarkers and patient stratification will allow achieving statistical significance on smaller patient populations or making more robust decision.
This type of AI is easy to implement in a fragmented manner or alongside of something which might look more familiar, such as a state-of-the-art method.
As soon as possible we should embark on the journey to use such AI in decision making in phase III studies as the methodology for extraction of biomarkers and assessment of efficacy.
This will of course require the consensus of regulatory bodies and all stakeholders, but the progress is being made every day.
AI and I, the Patient
The buzz phrase around bio-pharma companies developing novel treatments is ‘personalized medicine’ or ‘precision medicine ‘. With the core concept that we are all different and a diagnosis and treatment have to be personalized, there is an acute need for better understanding of a patient profile, or phenotype.
In 1990, when the first human genome was sequenced, it took 13 years at a cost of about $2.7 Billion dollars. Today this could be done in a matter of days at cost a few hundred dollars. As this data becomes more readily accessible, it is important to deploy robust data analysis to identify most efficacious and tolerable treatments at the patient level across populations, this only possible through AI and sight into data.
The scope of AI here is vast and includes
- prediction of cancer type across populations through genomes,
- automatic of personalized target for success of a treatment at a patient level,
- identifying the right dosing profile based on genomes and patient statistics and more.
This will revolutionize the drug development, right up from discovery, assessing libraries of thousands of compounds to assess which ones are most likely to succeed, through trial logistics, recruitment of patients based on genome and relevant characteristics, through to post-surveillance, what are the criteria that most influence if a patient will have an adverse response and acting in real time to address this.
These AI driven insights are different from traditional analytics, in both the sheer volume of data utilized, and the types of data incorporates as well as the use of a robust model which gets better as a product of more data and time.
To conclude, AI in either form provides a powerful mechanism of improving the business performance and decision power.
With a global clinical trial success rate of 9.6%, AI will generate value through savings in efficiency and centralization. As we, as a company are thinking about strategic use of imaging in the trials, we see how quantitative imaging biomarkers provide invaluable insights into the compounds and reveal those that are most likely to succeed at much earlier timepoint and smaller cohorts. This happens pretty much in every indication, but of course has the most value when developing advanced therapies.
This is a link to our nearly 2000 publication library, where you can find the indication of interest and most likely an AI driven methodology we used to assess the treatment efficacy: https://wp1.ia-grp.com/publications/
Through partnerships with biotech and pharma companies, we already were able to demonstrate the true value of advanced imaging assessment, AI-driven biomarkers and the disruptive potential of integrating AI in the drug development process.
As you are now thinking of embarking on an AI initiative, speak to our expert team @IAG, Image Analysis Group (wp1.ia-grp.com) to ensure that you select the right technology perform, optimal AI approach and data strategy to achieve your goals.
In order not to waste valuable time and resources for the drug developers and our patients, it is important to set the right expectations from the very start.
Author: Dr. Olga Kubassova, MMath, PhD, IAG, Chief Executive Officer
Mathematician with 10+ years expertise in actively managing innovation in life science companies, asset positioning, strategy and capital rising. Extensive expertise and domain knowledge in identifying innovative approaches to clinical research, disruptive technology platforms and novel therapeutics. Olga is a renowned healthcare innovator and biotech investor, with passion for improving people’s health. She is an active researcher, published author, scientific adviser to the governments, VC funds and funding bodies. Olga’s ambition is to bring truly disruptive technologies, artificial intelligence and best of machine learning to clinical practice and research, while expanding IAG’s footprint and partnerships.