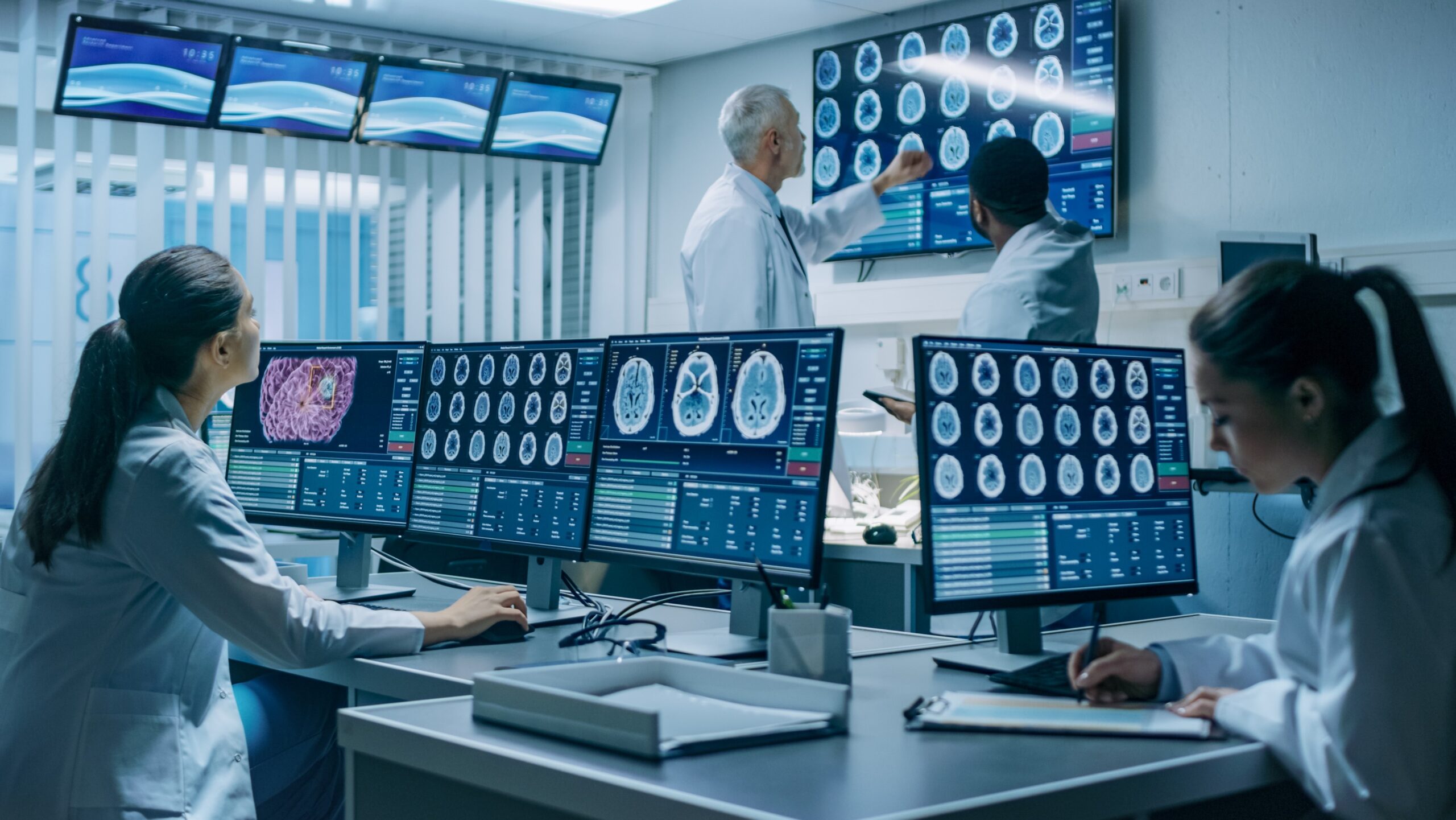
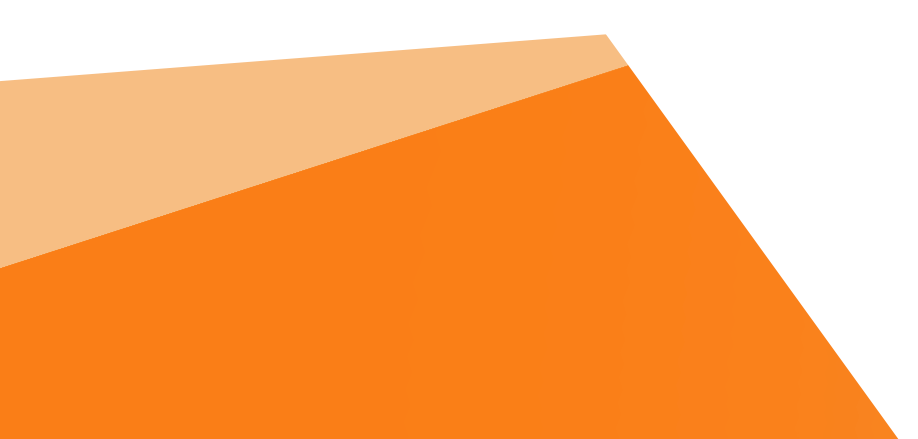
Post-Covid Era of Bio-Pharma R&D
We have no doubt that with efforts of @Lilly, @Gilead, @Pfizer and many other capable players we will find vaccine for #Covid19. While the world is focused on the #Covid, the cures for #cancer, #pain, #osteoartritis, #alzeimers and many diseases are much needed in our society.
These will come from small to mid-size #biotechs, who fearlessly innovate and despite everything come out with breakthroughs which change our lives and the lives of our loved ones.
In the time of #Covid19 and our economy slow down, for the biotech companies it is vital to continue generating the data and sharing positive news to support their evaluations and investors’ confidence.
How to ensure that #investment deal flow doesn’t slow down and how a small biotech stays in business?
This piece highlights our thoughts on how to prepare for a new era of #bio-#pharma R&D.
Generate Data while the Trial is Paused
For those biotech and pharma, who are not able to help the world by working on Covid-19, the data from their existing trials becomes the vital source of information. As the trials’ recruitment slows down, with the nurses and hospitals prioritizing Covid’s victims, each company has an opportunity to pause and understand their already existing data, e.g. retrospective data analysis.
This could be done with a deeper dive into #imaging, which is often left unattended till the end of the trial. The multi-omics or radiomics approaches can help with predicting of trial outcomes based on the early #CT or #MRI data. Patient stratification can be supported by linking the baseline data and the early responses.
Working through the retrospective data could be a way to bring to light further clarity on MoA of complex treatments.
Having clarity on the patient response and being able to link the patient phenotype to the first instances of response / non-response will allow for much better planning of the next steps of the trial.
Plan to reduce the Trial Budgets
As many trials are currently in the design stage, we must assume that the budgets for them will be reduced, if the economy slows down. Thus, it is critical to choose the optimal endpoints and patient populations to reach statistical significance with a smaller number of patients available.
This task is difficult because there is no clear path of getting to the right answer. It is easy to look at what has been done in terms of efficacy and efficacy endpoints 10-20 years ago and just do the same. Not surprisingly, this will not be a winning strategy.
With the latest developments in #imaging modalities, #AI and #MachineLearning, we are living in an era of unprecedented precision, patient phenotyping and early efficacy focused radiomics.
It is risky to rely on the past experience alone and skip deployment of R&D risk-lowering mechanisms.
Sub-optimal choice of imaging biomarkers and protocol designs will lead to higher costs of the trial and delays associated with patient recruitment.
We would advise any of our biotech or pharma partners, prior to the study start, to conduct in-depth market analysis to understand the range of efficacy endpoints achieved in phase II and phase III trials in this therapeutic area.
Think about designing smaller targeted studies for a coherent population. Compliment these, with the sub-studies, which might involve much more advanced imaging to reveal efficacy earlier or allow to stop the trial earlier. Partner with CROs who can engage sites in the right geographies to ensure speedy enrolment.
Ensure that there is the right technology platform in place so that you have a real time control over the data quality and trial performance.
Focus on the Right Patients
There is tremendous inter-patient variability in the responses to even highly efficacious treatments. This is a great source of frustration for our clinicians, which led to development of “precision medicine”.
However, this approach will only succeed if the characteristics of individual patients or subgroups of patients that influence the response to a specific treatment are captured and utilized effectively.
The challenge is to identify the measurable phenotypic characteristics of patients that are most predictive of individual variation in treatment outcomes, and the measurement tools that are best suited to evaluate these characteristics.
We see that combination of #imaging with clinical data can reduce a subjective assessment. When used at the design stage of the trial, this becomes a gold-thread through the entire process of the clinical development, ensuring that we remain objective in any decisions and not relying on patient or clinician reported outcomes exclusively.
Make Go / No Go Decisions Faster
With the use of advanced methods for the trial data assessment, we can see and measure treatment efficacy early at much shorter intervals. Surrogate endpoints based on CT or MRI scans in many trials focused on #rheumatic diseases or #oncology are the norm.
As the number of trials demanding patients increases, it is critical to give create a feedback loop to the sites and Physicians-Investigators to share the baseline patient data and early responses. This will help motivating patients and monitor someone on a high-risk treatment trial.
We expect that integration of #imaging into the trials will help maximizing clinical outcomes and chances of success through better patient retention and faster decisions.
Go Virtual
From site monitoring to data collection, from investigator meetings to educational webinars. This is the time and place to reduce the number of flights and go virtual.
What should become the norm are simple actions line sending data to the cloud and getting a real-time response, using predictive analytics and tele-radiology.
As we are coming into the new era of pharma R&D, we need to put in place rules and laws around data security and data sharing.
The governments should empower the patients and researchers to share the data and learn faster from global patient databases, national registries, from world-wide open access information centres.
Patients should not be afraid to give their data for research, to support each other and the industry. In return the industry will move faster, reducing the costs of R&D and subsequently failures. This should help reducing the costs of medicines when we see the successes.
At @IAG, we begin every study with integrating data flows to ensure interoperability of disparate systems and integration of data sources. Through the cloud data management platform, we need to eliminate data silos by continually harnessing all of the information in a trial from all of the data sources — sites, investigators, previous trials and sub-studies.
Real-time access to the insights from disparate data sources drive fact-based decisions and reduce prevalent risk factors in clinical studies.
Already today, in many of our latest studies, we automate a complex patient eligibility calculation after aggregating live data from multiple disparate data sources in order to immediately decide the right patients to enrol. In rheumatology trials, we bring together imaging scoring systems to correlate them to histology and make sure the patient’s pain is no longer a hidden undefined variable. In oncology, we can predict overall survival in ½ the time of the trial length through advanced imaging.
Tomorrow, we will need to do this even faster, in every trial. This will change our understanding of the disease and the speed of patient response.
This will give energy to our R&D efforts and a renewed momentum to pharma R&D