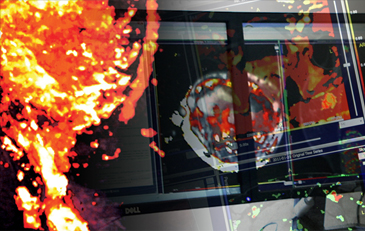
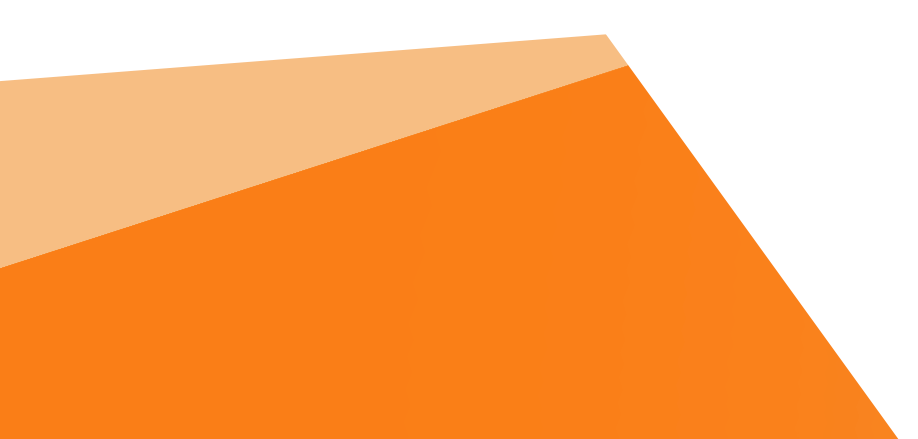
Power of Radiomics: Can We Predict Treatment Response to Immunotherapy?
Recent advances in artificial intelligence (AI) computational power and techniques have created a new area of research called Radiomics. Radiomics is defined as the high throughput extraction of quantitative features or texture from medical images.
Using medical images for tissue classification is a promising non-invasive method for phenotyping and treatment response prediction.
Radiomics features provide information about the grey-scale patterns, inter-pixel relationships, shape, and spectral properties of regions of interest, lesions in a medical image. These features can be further used to develop computational models using machine learning algorithms that can serve as a tool for precision medicine and treatment guidance [1].
A typical radiomics pipeline consists of the following modules:
1) image acquisition and pre-processing,
) tumour segmentation,
3) feature extraction,
4) feature selection,
5) predictive model generation.
Medical images include computed tomography (CT), magnetic resonance imaging (MRI) and positron emission tomography scans. During the pre-processing stage, algorithms reduce differences in the images for a more robust radiomics feature set. Tumour segmentation results in a clearly identified lesion in the image.
It is conventionally performed by an expert radiologist; however novel approaches have shown the possibility of automatization using deep neural networks. The most important part of a radiomics pipeline is the feature extraction and selection. Features are image characteristics, such as semantic features, first-order statistical features, shape, and volumetric features, texture-features.
Feature selection is the task of defining a subset of the most discriminant features. The final step is to define a prediction model for clinical outcomes with a few selected features. Machine learning provides various methods to achieve this goal. Typically, supervised, semi-supervised, and unsupervised learning are fundamental strategies used according to the different levels of available clinical outcomes.
Applications in drug development include early immunotherapy treatment response assessment. Features extracted from baseline pretherapy CT scans have been recently identified as a potential independent a predictor of response to Nivolumab and Pembrolizumab in advanced NSCLC [2,3].
Clinical biomarkers such as tissue-based CD3 count and PD-L1 expression showed high correlation with radiomics expression. Radiomics could predict highest overall survival in high CD3 and low PD-L1 expression cohorts [2]. Further, CT-derived texture parameters were shown to predict overall survival (OS) and progression-free survival (PFS), assessed by iRECIST criteria, in patients with NSCLC treated with first line Pembrolizumab [3].
The use of AI in the form of Radiomics is an area of research for IAG.
We actively work with our biotech, pharma and academic partners to implement novel methods in clinical trials to increase the scientific value of the study and accelerate the drug to market process. If you are planning or running a trial that may benefit from advanced imaging, reach out to imaging.experts@ia-grp.com
- V. Parekh and M. A. Jacobs, “Radiomics: a new application from established techniques,” Expert Rev. Precis. Med. Drug Dev., vol. 1, no. 2, pp. 207–226, 2016.
- Velcheti V, Alilou M, Khunger M. Changes in computer extracted features of vessel tortuosity on CT scans post-treatment in responders compared to non-responders for non-small cell lung cancer on immunotherapy. J Clin Oncol. 2017;35:11518–11518.
- Zerunian, M., Caruso, D., Zucchelli, A. et al. CT based radiomic approach on first line pembrolizumab in lung cancer. Sci Rep 11, 6633 (2021). https://doi.org/10.1038/s41598-021-86113-5
——————————-
If you are planning a new clinical trial, this is where we can help to think through the trial design, optimal use of imaging biomarkers, use of cloud platform for imaging data central review and use of AI – to assess complex manifestations of the new treatments. Email to: imaging.experts@ia-grp.com
About Image Analysis Group (IAG)
IAG, Image Analysis Group is a unique partner to life sciences companies. IAG leverages expertise in medical imaging and the power of Dynamika™ – our proprietary cloud-based platform, to de-risk clinical development and deliver lifesaving therapies into the hands of patients much sooner. IAG provides early drug efficacy assessments, smart patient recruitment and predictive analysis of advanced treatment manifestations, thus lowering investment risk and accelerating study outcomes. IAG bio-partnering takes a broader view on asset development bringing R&D solutions, operational breadth, radiological expertise via risk-sharing financing and partnering models.
Learn more: ia-grp.com
Reach out: imaging.experts@ia-grp.com
Follow the Company: Linkedin